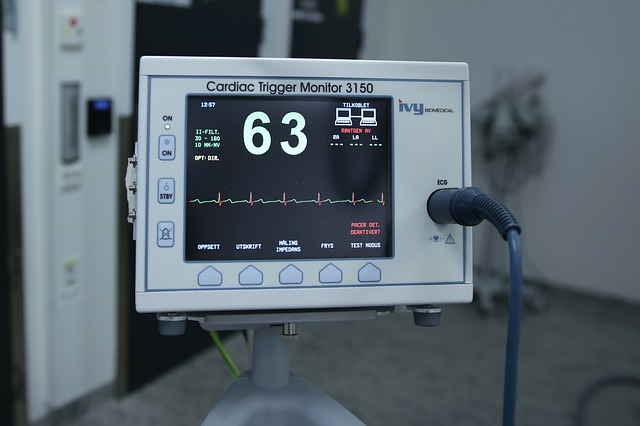
An Artificial Intelligence enabled ECG can now detect heart disease which otherwise was not possible through a normal electrocardiogram. Its another step towards better detection of heart disease like Hypertrophic cardiomyopathy (HCM).
This recent research by scientist at Myoclinic was published in the Journal of the American College of Cardiology and they found a unique blend of Artificial Intelligence and ECG to screen the Hypertrophic cardiomyopathy (HCM), an uncommon but important cause of sudden cardiac death.
ECG and AI
ECG or electrocardiogram is used by doctors to record the electrical activity of the heart there by detecting otherwise any abnormal electrical signal. Abnormal signal gives a clue about the possible heart disease.
But, researchers were curious to develop an artificial intelligence approach for the detection of HCM which otherwise was not possible through normal ECG.
Interestingly, there is another research that has developed an AI enabled ECG capable of predicting the hidden information about our overall health.
The present research was specific to detecting HCM by AI enabled ECG.
2,448 patients with HCM diagnosis were recruited
Researchers wanted to train the convolutional neural network (CNN) an Artificial Intelligence tool for which they needed person diagnosed with HCM.
So, researchers recruited 2,448 patients with a verified HCM diagnosis and 51,153 non-HCM age- and sex-matched control subjects.
They trained convolutional neural network (CNN) by collecting data from all the participants validated using 12-lead.
Then they agin tested this trained CNN on another cohort of patient (612 patient with HCM and 12788 controls) to find out its capability to detect this heart disease HCM.
Also read: Heart Attack: Better prediction with New Artificial Intelligence enabled tool
AI enabled ECG performed high diagnostic performance
After training and validation, the area under the curve (AUC) of the CNN in the validation dataset was 0.95 (95% confidence interval) at the optimal probability threshold of 11% for having HCM.
When applying this probability threshold to the testing dataset, the CNN’s AUC was 0.96 (95%) with sensitivity 87% and specificity 90%. In subgroup analyses, the AUC was 0.95 (95%) among patients with left ventricular hypertrophy by ECG criteria and 0.95 (95% CI: 0.90 to 1.00) among patients with a normal ECG.
The model performed particularly well in younger patients (sensitivity 95%, specificity 92%). In patients with HCMÂ with and without sarcomeric mutations, the model-derived median probabilities for having HCM were 97% and 96%, respectively.
Journal reference:
Detection of Hypertrophic Cardiomyopathy Using a Convolutional Neural Network-Enabled Electrocardiogram Wei-Yin Ko, Konstantinos C. Siontis, Zachi I. Attia, Rickey E. Carter, Suraj Kapa, Steve R. Ommen, Steven J. Demuth, Michael J. Ackerman, Bernard J. Gersh, Adelaide M. Arruda-Olson, Jeffrey B. Geske, Samuel J. Asirvatham, Francisco Lopez-Jimenez, Rick A. Nishimura, Paul A. Friedman, Peter: DOI: 10.1016/j.jacc.2019.12.030